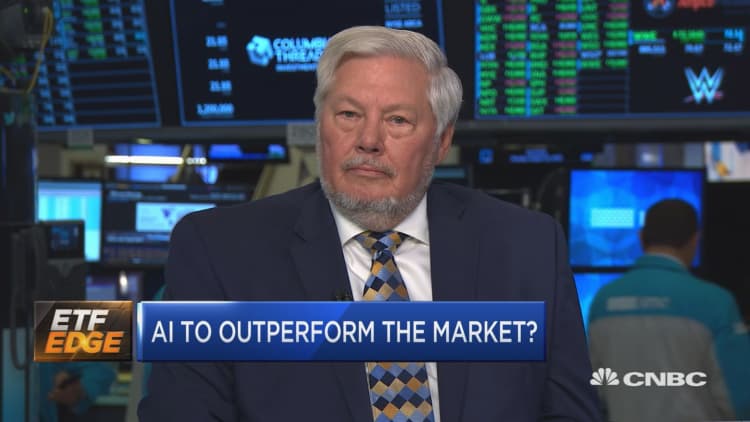
Artificial intelligence and machine learning could be the next frontier for ETFs to outperform the market.
So says Robert Tull, President of ProcureAM, an innovative exchange-traded product firm and wholly owned subsidiary of Procure Holdings.
A veteran in the business, Tull has been involved in the ETF industry for decades, creating more than 400 ETFs across 18 different countries. Now, he's looking at new ways to beat the market by using big data as raw material, combined with machine learning, to build ETF portfolios that could potentially outperform active management — even actively managed ETFs.
"Active management has been out there for a long time, underperforming," he said on CNBC's "ETF Edge." "They haven't found a solution yet, and I think the technology that I've run into is going to help the marketplace today."
The key to this new technology is ensemble analytics, a type of methodology that uses multiple learning algorithms to better predict performance.
"The technology's been around for years," Tull said. "It's just never moved into the asset management space, so [it's about] getting data collected, running permutations against it and then really focusing on the best of the best selection that's diversified."
In other words, instead of picking individual stocks, Tull aims to make smart beta even smarter by extracting data from other ETFs and attempting to build the smartest of them all.
Smart beta involves the use of a rules-based system, or a series of factors, for selecting investments to include in a fund's portfolio. These funds are governed by a specific set of rules and are typically weighted differently from the traditional market cap-based weighting scheme.
Goldman Sachs has done just this with its ActiveBeta U.S. Large Cap Equity ETF (GSLC), which looks at four different factors — value, momentum, high quality and low volatility — and weights them accordingly.
The index — which counts Microsoft, Apple, Amazon, Johnson & Johnson and Facebook as its top five largest holdings — has fared well in 2019, up about 15%.
Tull has taken this same model, used it as a benchmark and applied his own secret sauce of big data analytics and AI to eliminate three out of the five stocks — Microsoft, Apple and Johnson & Johnson — through mathematics and modeling, giving Amazon and Facebook each a 5% weighting.
The thinking behind this is that the collective wisdom of every smart beta ETF out there — including Goldman's — is better than the mindset of any individual set of stock pickers.
"You're going to add the data to it that, quite frankly, a human brain just can't digest," said Tull.
So, the key question becomes, is there any evidence that machine learning can actually outperform when it comes to picking stocks?
Dave Nadig, who runs ETF.com, says there is.
He points to the AI Powered Equity ETF (AIEQ), which has risen 17%, besting the S&P 500 this year. The fund, run by Equbot, uses both A.I. and IBM Watson to find opportunities in the market.
"I think this is the next generation, frankly, of financial product development," said Nadig. "Machine learning sounds big and scary, but all it is, is really just taking data and things you already know, how things perform, to generate rules – as opposed to hiring a bunch of CFAs to come up with those rules about what you're going to buy and sell based on fundamentals."
Tull added that while his A.I.-powered programs are still in the early stages of development, a number of companies have already shown interest in the idea.
"I can tell you that there are multiple insurance companies that have done their due diligence tests who are signing up," he added. "We have derivatives desks who are signing up, so I think they [along with the data] have convinced me that this is the future."